We do not have a control group! Or, do we?
When assessing the effect of an action with an experiment, a typical workflow will not only define the technical steps for getting results; it will also introduce a checklist of conditions that should hold if we want the results to be reliable. This includes things like proper randomization (and covariate balance), perfect compliance, checks for sample ratio mismatch and more.
Of fundamental importance is the existence of a well-defined, properly-sized control group whose results we will be comparing to those of the units that were treated. Normally, holding back a set of units from receiving treatment is not a big deal - as this is most of the time temporary and the stakeholders are not missing out on much.
A case where the business perspective differs is that of marketing campaigns. Teams may be hesitant to deprive users of marketing communications - as this might be thought of as negative for the business1, and campaigns sometimes simply cannot wait. As a result, campaign experiments might be highly imbalanced (i.e., the treatment group is much larger than control). This is not a big problem, as valid inference is still possible - with the caveat of lower statistical power.
What happens however if we push this paradigm to the extreme by simply contacting all eligible users and therefore entirely omitting a control group? This is a case I recently encountered, finding myself in a challenging situation: wanting to make causal inferences without a control group.
Coming from a background where interventions in the data were carried out by complex actors (nature / humans), I had limited hope in successfully estimating the treatment effect. Companies however may be complex in how they operate, but they are simple in terms of the goals they pursue and rational in how they go about achieving them.
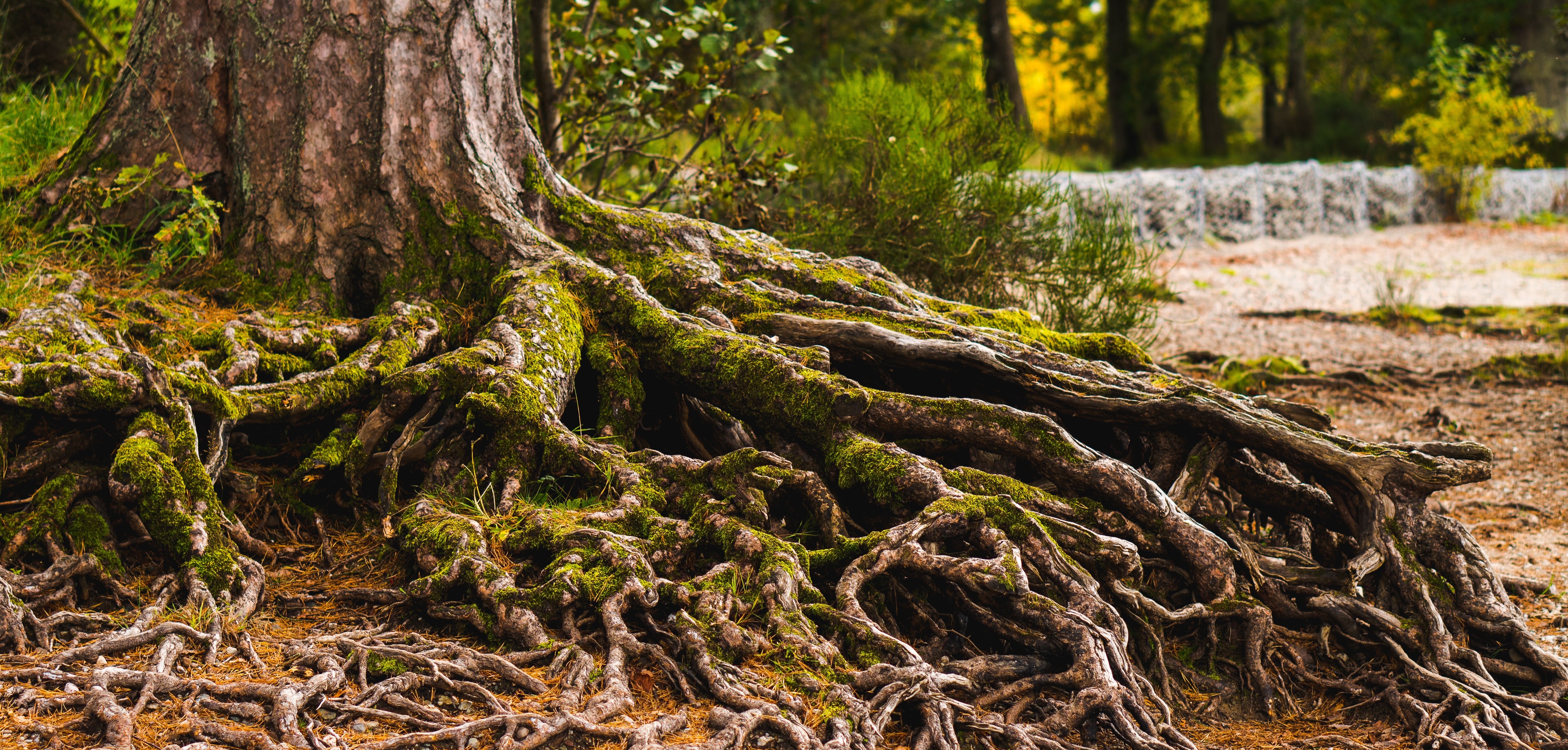
The solution to my problem came from a topic much discussed and a power that keeps on giving: business understanding. In the case of the campaign, while no randomization happened and no control group was established, the audience was defined based on a sharp threshold of some unit data. This enabled the implementation of a custom Regression Discontinuity Design that made possible the estimation of a Local Average Treatment Effect. While quasi-experimental, this estimate was ultimately good enough for campaign evaluation purposes, and in retrospect allowed all relevant units to be contacted with perfect timing.
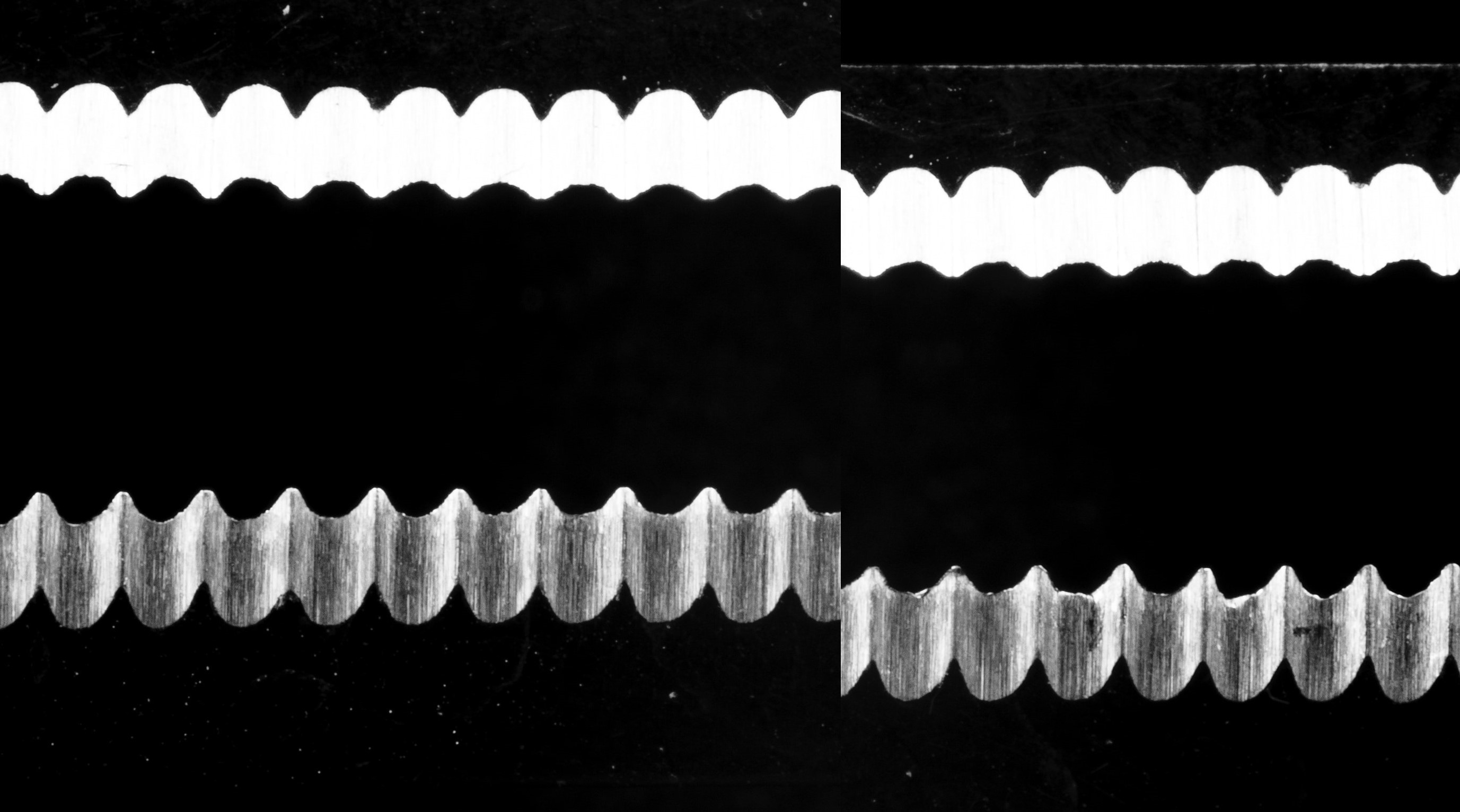
This was a lesson learned for me too: causal inference when the treatment assignment logic is known may still be possible in the absence of a control group - or, alternatively, a control group may still exist outside the “experiment”. Life is easier if we understand the treatment assignment mechanism, even if no randomization is employed2.
-
If short-term revenue is the north star metric - which should not necessarily be Kohavi & Longbotham 2011 ↩
-
A relevant post can be found at the Linkedin profile of Matheus Facure, author of “Causal Inference for the Brave and True”. ↩